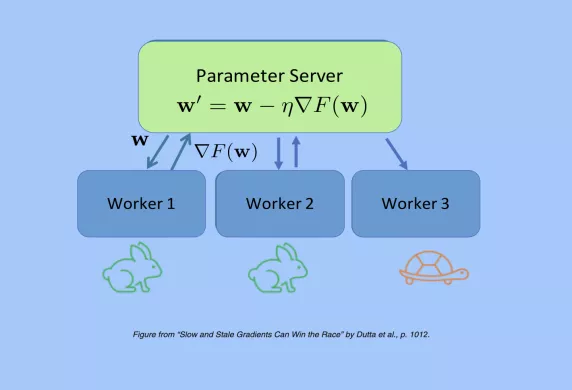
The CFP for JSAIT's 6th Special Issue. The intersection of information theory and computing has been a fertile ground for novel, relevant intellectual problems. Recently, coding-theoretic techniques have been designed to improve performance metrics in distributed computing systems. This has generated a significant amount of research that has produced novel fundamental limits, code deigns and practical implementations. The set of ideas leveraged by this new line of research is collectively referred to as coded computing. This special issue will focus on coded computing, including aspects such as tradeoffs between reliability, latency, privacy and security.
Generalization error bounds are essential for comprehending how well machine learning models work. In this work, we suggest a novel method, i.e., the Auxiliary Distribution Method, that leads to new upper bounds on expected generalization errors that are appropriate for supervised learning scenarios. We show that our general upper bounds can be specialized under some conditions to new bounds involving the $\alpha $ -Jensen-Shannon, $\alpha $ -Rényi $(0\lt \alpha \lt 1)$ information between a random variable modeling the set of training samples and another random variable modeling the set of hypotheses. Our upper bounds based on $\alpha $ -Jensen-Shannon information are also finite. Additionally, we demonstrate how our auxiliary distribution method can be used to derive the upper bounds on excess risk of some learning algorithms in the supervised learning context and the generalization error under the distribution mismatch scenario in supervised learning algorithms, where the distribution mismatch is modeled as $\alpha $ -Jensen-Shannon or $\alpha $ -Rényi divergence between the distribution of test and training data samples distributions. We also outline the conditions for which our proposed upper bounds might be tighter than other earlier upper bounds.